Your cart is currently empty!
Demystifying the 1.5 Sigma Shift
The subject of internal performance metrics often underestimating customer issues has been a long-standing debate in the field of Six Sigma. The 1.5 sigma shift is one such topic that has sowed seeds of confusion, and often annoyance, amongst many. As someone who has closely examined this aspect for years, I believe it is high time to lift the shroud of mystery surrounding this “statistical correction” and lay out its true purpose clearly.
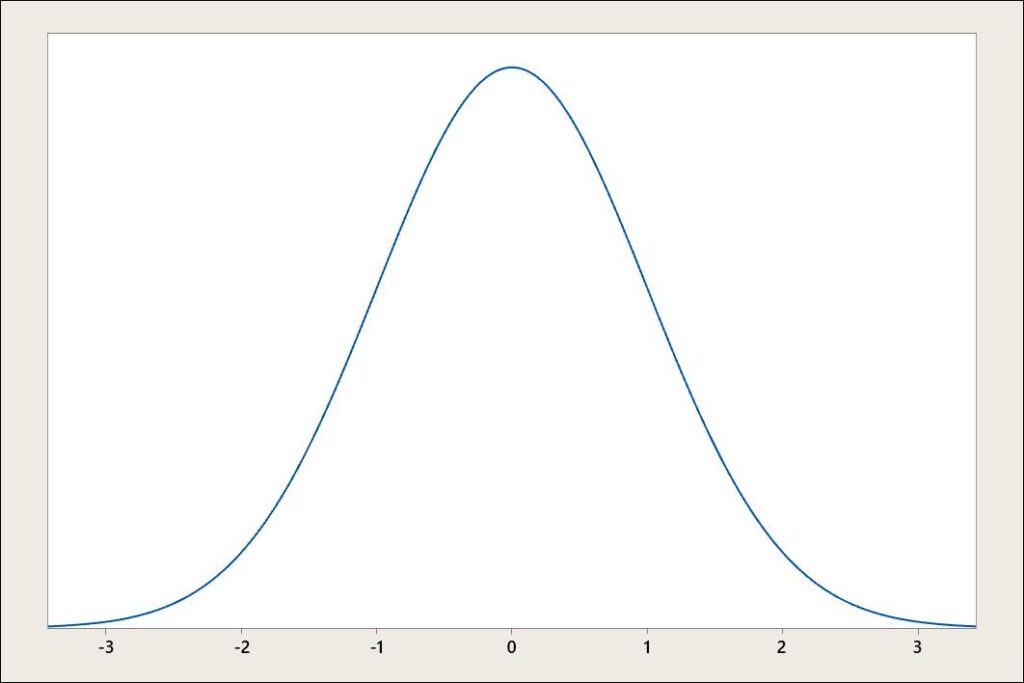
The standard normal distribution curve is our starting point. Widely studied and charted, it is a close approximation of many natural systems. Although the distribution stretches indefinitely in both directions, graphical representations usually display the range between -3 to +3 standard deviations, capturing 99.73% of the data. Prior to the advent of Six Sigma, all quality calculations were grounded in this distribution without any alterations.
Consider a scenario where customers express dissatisfaction when hold times go beyond 15 minutes. If the hold times average 12 minutes, with a standard deviation of one minute and are perfectly normally distributed, the 15-minute specification sits three standard deviations above the mean. Using the area of a normal distribution beyond +3 standard deviations, we can predict that 0.135% of customer hold times will surpass the specification.
In contrast, Six Sigma tweaks this procedure by making a 1.5 sigma “adjustment” to the calculated mean before estimating the percentage of specifications. For the hold time example, we would calculate with an average hold time of 13.5 minutes, not the actual 12 minutes. This change results in an estimated 6.68% exceeding the specification.
A common adage among engineers and scientists is, “All models are wrong, but some models are useful.” While the conventional normal model is indeed flawed, it often remains functional. The question then is, does the 1.5 sigma adjustment yield a model that is more effective than the traditional one? I believe it does. The traditional model, while simplifying reality, tends to overly polish things from our perspective, making them appear rosier than they are to our customers.
Various assumptions underline the hold-time example. Perfect normality for hold times, sampling error, the definition of “hold time” (customer-perceived hold time vs. clock time), and considerable variability among customers, to name a few. In a production context, oversimplifications may include estimating sigma based on short-term variation, taking measurements on unused product, not factoring in shipping and handling effects, overlooking environments to which the product will be exposed, and a lack of understanding of customers’ requirements. The 1.5 sigma shift is a corrective mechanism that factors in elements excluded in our model of reality.
Process Sigma Level | Process PPM | Process Sigma Level | Process PPM |
---|---|---|---|
6.27 | 1 | 4.66 | 800 |
6.12 | 2 | 4.62 | 900 |
6.00 | 3.4 | 4.59 | 1,000 |
5.97 | 4 | 4.38 | 2,000 |
5.91 | 5 | 4.25 | 3,000 |
5.88 | 6 | 4.15 | 4,000 |
5.84 | 7 | 4.08 | 5,000 |
5.82 | 8 | 4.01 | 6,000 |
5.78 | 9 | 3.96 | 7,000 |
5.77 | 10 | 3.91 | 8,000 |
5.61 | 20 | 3.87 | 9,000 |
5.51 | 30 | 3.83 | 10,000 |
5.44 | 40 | 3.55 | 20,000 |
5.39 | 50 | 3.38 | 30,000 |
5.35 | 60 | 3.25 | 40,000 |
5.31 | 70 | 3.14 | 50,000 |
5.27 | 80 | 3.05 | 60,000 |
5.25 | 90 | 2.98 | 70,000 |
5.22 | 100 | 2.91 | 80,000 |
5.04 | 200 | 2.84 | 90,000 |
4.93 | 300 | 2.78 | 100,000 |
4.85 | 400 | 2.34 | 200,000 |
4.79 | 500 | 2.02 | 300,000 |
4.74 | 600 | 1.75 | 400,000 |
4.69 | 700 | 1.50 | 500,000 |
*Assumes that in the long term the process could drift by ±1.5σ (PPM=errors or defects per million opportunities)
The table illustrates the equivalent yields obtained when considering the 1.5 sigma shift. Use the table as per the following instructions:
Measurement Data
When using measurement data, calculate the average and standard deviation. Then, determine how many standard deviations separate the mean from each specification. Locate the corresponding PPM level in the table. Combine the PPM values for the lower and upper specification and find the process sigma level in the table.
Example
For a hole diameter specification of 0.990 in. to 1.010 in. with an average of 1.005 in., and sigma = 0.0025 in., standard deviations to upper specification: 2 (PPM = 307,400 by interpolation); standard deviations to lower specification: 6 (PPM = 3.4 or approximately 0). Therefore, expected errors = 307,400+, indicating a process sigma level of 2.
Attribute Data
For data derived from observed error or defect counts, convert the count to PPM using the formula PPM = 1,000,000 x (error count/error opportunities). Find the corresponding process sigma level using the PPM value.
Example
If 400 units were shipped and 10 were returned as defective, PPM = 1,000,000 x (10/400) = 25,000, leading to a process sigma level of 3.47 by interpolation.
In the counts example, we computed a process sigma level of 3.47 based on a 2.5% defective rate. On checking Z = 3.47 in a normal table, we find that the projected defective rate is 0.03%, significantly better than the observed result. Many statisticians view this as unjustly claiming “credit” for 1.5 sigma. However, this perspective arises from a limited statistical interpretation of the 1.5 sigma shift. When viewed as an endeavor to align our model more closely with reality, the interpretation shifts: The customer observed 2.5% failures during actual product usage, which aligns with an internal failure rate estimate of merely 0.03%.
It’s a recognized fact that our internal performance metrics often fall short of reflecting the issues experienced by our customers. By offering a simple rule of thumb that corrects for the oversimplification inherent in the traditional model, the 1.5 sigma shift adjustment allows us to view our products and services through our customers’ lens. Like all models, this too is imperfect. However, many companies have found it to be a more valuable tool in steering their business compared to the traditional model.