Your cart is currently empty!
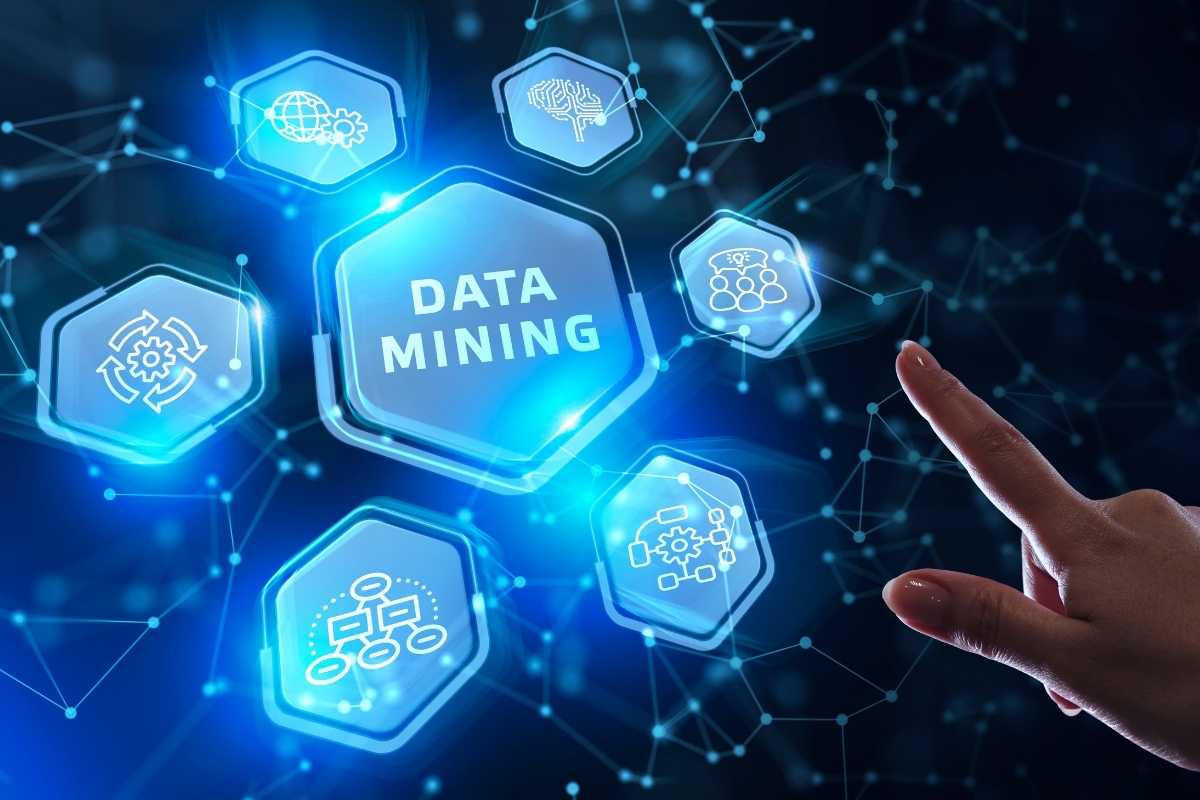
Six Sigma, Data Mining and Dead Customer Accounts
In an interview with Quality Digest, Joseph M. Juran pointed out that quality needs to “scale up” if it is to remain a viable force in the next century. In other words, quality must spread beyond its traditional manufacturing base.
A major opportunity to do just that now exists, as Six Sigma enjoys a resurgence of interest among quality professionals and “data mining” is the hot topic among information systems professionals. Six Sigma involves getting 10-fold improvements in quality very quickly; data mining uses the corporate data warehouse, the institutional “memory,” to obtain information that can help improve business performance.
The two approaches complement each other, but have differences as well. Data mining is a vaguely defined approach for extracting information from large amounts of data. This contrasts with the usual small data set analysis performed by quality engineers and statisticians. Data mining also tends to use automatic or semiautomatic means to explore and analyze the data. Again, this contrasts with traditional hands-on quality applications, such as control charts maintained by machine operators. Data mining for quality corresponds more closely with what Taguchi described as “off line” quality analysis. The idea is to tap into the vast warehouses of quality data kept by most businesses to find hidden treasures. The discovered patterns, combined with business process know-how, help find ways to do things better.
Data-mining techniques tend to be more advanced than simple SPC tools. Online analytic processing and data mining complement one another. Online analytic processing is a presentation tool that facilitates ad hoc knowledge discovery, usually from large databases. Whereas data mining often requires a high level of technical training in computers and statistical analysis, OLAP can be applied by just about anyone with a minimal amount of training.
Despite these differences, both data mining and OLAP belong in the quality professional’s tool kit. Many quality tools, such as histograms, Pareto diagrams and scatter plots, already fit under the information systems banner of OLAP. Advanced quality and reliability analysis methods, such as design of experiments and survival analysis, fit nicely under the data mining heading. Quality professionals should take advantage of the opportunity to share ideas with their colleagues in the information systems area.
Real World Example
A bank wants to know more about its customers. It will start by studying how long customers stay with the bank. This is a first step in learning how to provide services that will keep customers longer.
This should be considered a quality study. The quality profession tends to focus too much on things gone wrong in identifying failures, then looking for ways to fail less. An alternative is to examine things done right, then look for new ways to do things that customers will like even better. This proactive, positive approach is a key to quality scale-up and a ticket into an organization’s mainstream operations.
This bank’s baseline study can also be viewed in the traditional failure-focused sense of quality. If customer attrition is viewed as “failure,” a host of quality techniques can be used on the problem at once. In particular, reliability engineering methods would seem to apply. If we look at creating a new account as a “birth” and losing an account as a “death,” the problem becomes a classic birth-and-death process perfectly suited to reliability analysis. Rather than using a traditional reliability engineering method like Weibull analysis, the following example uses a method from healthcare known as Kaplan-Meier survival analysis.
Survival analysis studies the time to occurrence of a critical event, such as a death or, in our case, a terminated customer account. The time until the customer leaves is the survival time. Kaplan-Meier analysis allows analysis of accounts opened by customers at any time during the period studied and can include accounts that remain open when the analysis is conducted. Accounts that remain open are known as censored because the actual time at which the critical event (closing the account) occurs is unknown or hidden from us.
Table 1 shows the first few database records (customer names are coded for confidentiality.) The database of 20,000+ records is large by traditional quality standards, but tiny by data-mining standards. The bank manager wishes to evaluate customer accounts’ lifespan. She also suspects that customers who use the bank’s Web banking service are more loyal. Figure 1 shows the survival chart for that data set.
Full Name | Date In | Date Out | City | Web User | Data Analyzed |
---|---|---|---|---|---|
David P | 26-Feb-18 | 28-Feb-18 | Sierra Vista | 0 | 28-Feb-18 |
David H | 26-Feb-18 | 11-Aug-18 | Sierra Vista | 0 | 11-Aug-18 |
Rick H | 29-Nov-15 | Tucson | 0 | 14-Feb-19 | |
Login P | 15-Feb-16 | Sierra Vista | 1 | 14-Feb-19 | |
Ron A | 26-Feb-18 | 21-Apr-18 | Sierra Vista | 0 | 21-Apr-18 |
William M | 26-Feb-18 | 2-May-18 | Sierra Vista | 0 | 2-May-18 |
Kevin F | 26-Feb-18 | 1-Jun-18 | Sierra Vista | 0 | 1-Jun-18 |
Andy N | 26-Feb-18 | 9-Sep-18 | Sierra Vista | 0 | 9-Sep-18 |
William P | 6-Aug-16 | Sierra Vista | 0 | 14-Feb-19 | |
Gary H | 20-Feb-16 | Sierra Vista | 0 | 14-Feb-19 | |
Stephen B | 26-Feb-18 | 28-Feb-18 | Sierra Vista | 0 | 28-Feb-18 |
Carl F | 27-Jun-16 | Benson | 0 | 14-Feb-19 |
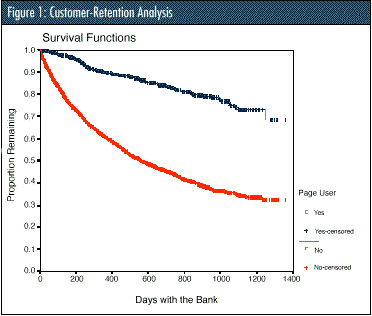
The information confirms the branch manager’s suspicion: Web users clearly stick around much longer than nonusers. However, the chart doesn’t tell us why they do. For example, Web users may be more sophisticated and better able to use the bank’s services without “hand holding.” Or there may be demographic differences between Web and non-Web users. The data was also stratified by the customer’s city, revealing many more interesting patterns and raising more questions.
An obvious question is what the bank might do to improve the overall retention rate; after all, nearly 70 percent of the customers left within 1,400 days of opening their account and, from a quality viewpoint, what “defect” could possibly be more serious than a customer leaving your business?
Lean Six Sigma professionals already have a full arsenal of tools and techniques for extracting information from data and knowledge from information. Long before information systems became popular, data helped guide continuous improvement and corrective action. Today, organizations are having an extremely difficult time finding qualified people to help them deal with the deluge of information. It’s time we let people in the nontraditional areas of the organization know that they have an underutilized resource right under their noses: the Lean Six Sigma professional.
3 responses to “Six Sigma, Data Mining and Dead Customer Accounts”
Interesting post, Tom.
Reliability Analysis is the Rodney Dangerfield of the industrial statistics world, it simply doesnโt get the respect it deserves.
We often evaluate our product as soon as it comes off the production line or after final inspection and deem it to be great or good or awesome, but the truth is most products should be evaluated for their ability to satisfy the customer after the product has been in service for 10 or 15 years. Statistical software has powerful Reliability features, but in my experience, they arenโt used very often, even by companies that routinely test to failure or proof test their product and have all that wonderful failure data and censored data at hand. Break or crush strength data is one example and there are many more.
Your article shows how Reliability Analysis can even be used in a financial context. Great stuff!
Well Tom, I think I jinxed myself. Iโve had a DVD player, a LED printer, a relatively new car tire and three LED light bulbs fail since I posted my comment.
I think this is more proof that companies need to pay more attention to improving their product reliability.
Very insightful article! The discussion on integrating Six Sigma principles with data mining to address dead customer accounts is both innovative and practical. The focus on using data-driven strategies to streamline processes and improve customer retention is crucial. The examples and methodologies provided are helpful for understanding how to implement these concepts effectively. It would be interesting to explore further how these techniques can be adapted for different industries or customer segments.
Read More:- soulilution.com
Leave a Reply