Your cart is currently empty!
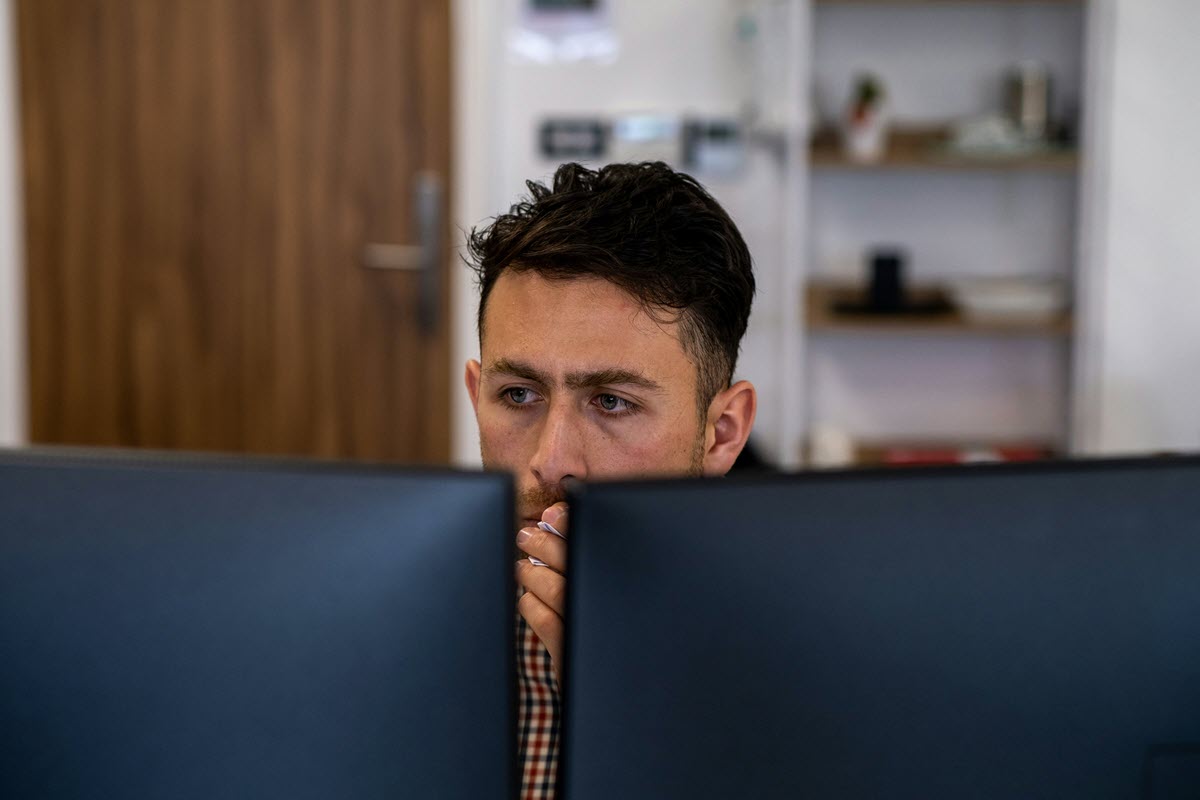
How Design of Experiments (DOE) Enhances Problem Solving in Six Sigma Projects
When we really get into the nitty-gritty of Six Sigma’s powerful methodologies, few tools are as remarkably impactful as Design of Experiments (DOE). Six Sigma is about eliminating guesswork and embracing data-driven decision-making, and nowhere is this more evident than in the application of DOE. In this post, Iโll show how DOE serves as a cornerstone in problem-solving within Six Sigma projects and share real-life examples that highlight its transformative impact.
The Role of DOE in Six Sigma: Turning Questions into Data-Driven Answers
In a world filled with variables and complexities, understanding the relationship between different factors can be rather challenging. Traditional problem-solving often relies on trial and error or gut instinct, but DOE shifts the paradigm by allowing us to systematically explore the relationships between variables in a process. It enables practitioners to identify which factors significantly influence outcomes and determine the optimal settings for improved performance.
Simply put, DOE is a structured statistical method that helps us make informed decisions about which factors truly matter when it comes to achieving process improvements. It helps Six Sigma teams move from “firefighting” to a proactive strategic approach to problem-solving.
Real-Life Example 1: Reducing Defects in an Injection Molding Process
Consider a manufacturer experiencing high defect rates in their plastic injection molding process, with common issues like warping, sink marks, and dimensional inconsistencies. The defects were costly, impacting both productivity and customer satisfaction. The team, armed with Six Sigma principles (and plenty of determination), turned to DOE to tackle this persistent problem.
Applying DOE: The team identified key process variables such as mold temperature, injection pressure, cooling time, and material type as potential contributors to the defect rate. By employing a DOE approach (which involves designing an experiment that systematically varies these factors across a range of values), they analyzed how each one influenced the defect rate.
Results: The DOE revealed that cooling time and injection pressure had statistically significant impacts on reducing defects while mold temperature and material type had minimal influence. By focusing on optimizing these two key variables (cooling time and injection pressure), the team reduced the defect rate by 30% within just weeks. This not only saved thousands of dollars in scrap costs but also significantly improved product quality.
Key Takeaway: DOE helped pinpoint critical factors impacting quality (allowing them to make data-driven adjustments) leading to dramatic reductions in defects. Without properly applying DOE methods, they might have continued wasting resources on irrelevant factors.
Real-Life Example 2: Enhancing Yield in Chemical Production Processes
In another instance, a chemical manufacturer faced significant challenges with low yield during a crucial production phase. Several steps, including temperature, reaction time, pH level, and catalyst concentration, were suspected to influence the final yield. Despite numerous attempts to adjust these variables independently, success remained elusive until…
Applying DOE: The team used fractional factorial experiments, which allowed them to evaluate multiple elements simultaneously without testing every possible combination. This approach was particularly useful given the large number of variables and the time-consuming nature of the production process.
Results: The experimentation revealed a strong interaction between reaction time and temperature, along with significant effects from pH levels on yields. Interestingly, the catalyst concentration, initially thought to be a major factor, had minimal influence. Armed with this data, the team adjusted the process parameters, ultimately increasing yields by 20% and saving substantial costs in raw materials and production time.
Key Takeaway: Utilizing DOE enabled the team to uncover interactions among various factors, leading to a holistic understanding of the process. This facilitated targeted improvements that resulted in substantial enhancements.
Real-Life Example 3: Enhancing Cycle Time in a Call Center
It’s not just the manufacturing sector that benefits from DOE; service industries can also see significant gains. A national call center was struggling with prolonged average handling times (AHT) for customer service calls, which led to increased operational costs and reduced customer satisfaction. Factors such as agent training, call routing methods, script structure, and software interface responsiveness were identified as potential contributors to the issue.
Applying DOE: The Six Sigma team applied DOE to analyze how these variables impacted AHT. They designed an experiment that adjusted elements like call routing methods, agent training levels, and script structure to observe how these changes influenced call handling time.
Results: The DOE analysis revealed that agent training and script structure had the most significant impact on reducing AHT, while changes to call routing methods and software interface speed were less influential than initially assumed. By focusing on enhancing agent training and refining script structures, the call center reduced average handling time by approximately 15%, resulting in considerable cost savings and improved customer satisfaction.
Key Takeaway: In this service industry scenario, DOE guided the team to focus on the factors that truly affected call handling time, enabling them to implement targeted solutions that delivered measurable improvements.
Why DOE is Invaluable in Six Sigma Problem Solving
The true power of DOE lies in its ability to reveal the relationships between process variables and outcomes. Unlike trial-and-error approaches, which can be inefficient, time-consuming (and let’s face itโfrustrating), DOE provides a structured, clear path for identifying and optimizing crucial factors. It enables Six Sigma practitioners to:
- Identify Key Drivers: DOE pinpoints the variables that have the most significant impact on process performance, allowing teams to focus their efforts where they matter most.
- Understand Interactions: Processes are rarely influenced by single factors; they often involve multiple interacting elements. DOE reveals how these variables interact, offering a deeper understanding of the process.
- Optimize Processes: By determining the optimal settings for critical variables, DOE enables teams to fine-tune processes and achieve the best possible outcomes.
- Save Time & Resources: With its structured approach, DOE eliminates the need for endless trial-and-error cycles, accelerating problem-solving and delivering results more efficiently.
Conclusion: Elevating Six Sigma Projects with Design of Experiments
Design of Experiments is much more than just a statistical toolโitโs a strategic approach that transforms how Six Sigma projects address problem-solving. By systematically exploring relationships between process variables, DOE enables practitioners to uncover hidden insights, optimize processes, and drive substantial improvements.
The real-world examples shared here demonstrate the pivotal role of DOE in reducing defects, enhancing yields, and improving service efficiency. These examples highlight the transformative potential of DOE across diverse industries. By incorporating DOE into your Six Sigma toolkit, you not only gain a deeper understanding of your processes but also unlock the ability to drive lasting, data-driven improvements.
As we often say, “Data doesn’t lie.” Design of Experiments helps us truly listen to what the data is telling us. When applied correctly, DOE becomes a guiding light on the journey toward operational excellence, providing clarity, direction, and the confidence to make decisions that lead to real, measurable success.
Incorporate Design of Experiments, master your process, and drive success. By embracing DOE, you take a decisive step toward mastering process complexities, solving problems with precision and efficiency, and transforming challenges into opportunities for breakthrough improvements.
One response to “How Design of Experiments (DOE) Enhances Problem Solving in Six Sigma Projects”
Great article
For those considering running designed experiments to optimize processes, be sure to consider Pyzdek Institute’s excellent workflow diagrams. They integrate measurement integrity studies, control point integrity studies and other statistical preliminaries with the latest experimental designs like A-Optimal, I-Optimal and Definitive Screening.
From my practical experience, following Pyzdek’s workflow results in lower cost of experimentation and better predictive models.
Leave a Reply