Your cart is currently empty!
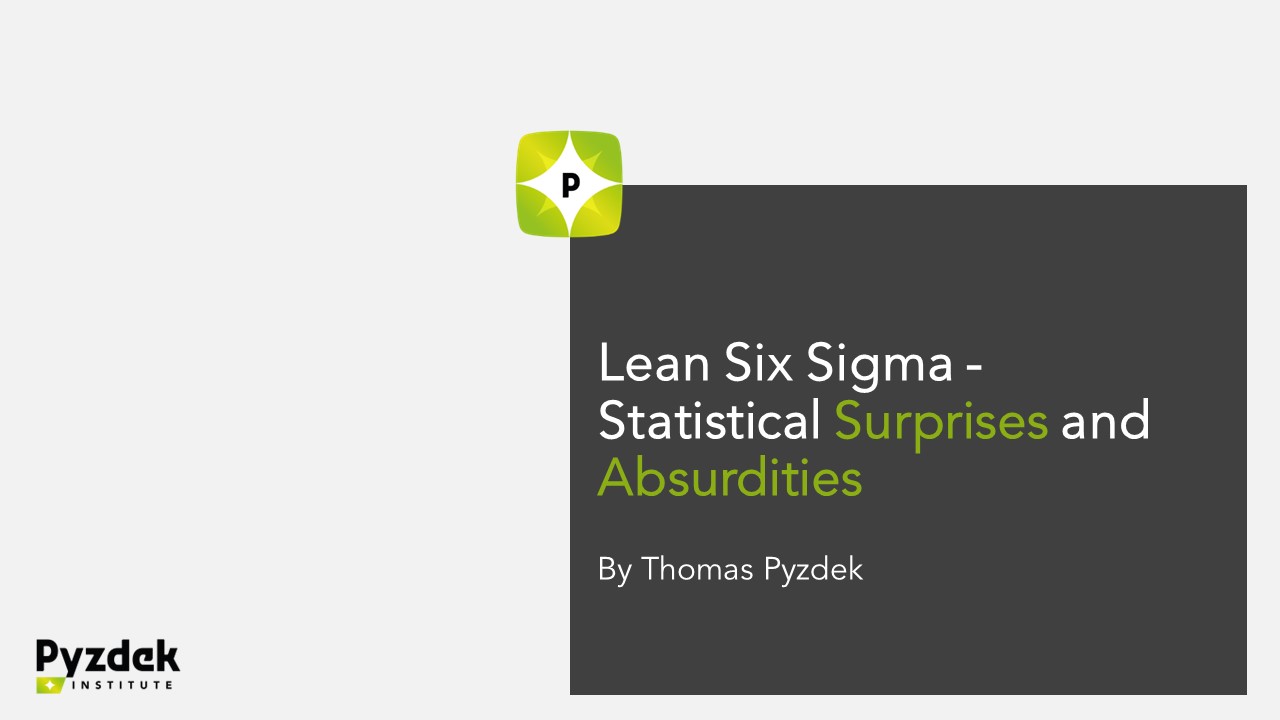
Lean Six Sigma – Navigating Statistical Surprises and Absurdities
We’re pleased to introduce an enlightening video presentation by Lean Six Sigma expert, Thomas Pyzdek. This presentation, titled “Lean Six Sigma – Statistical Surprises and Absurdities,” dives into the potential traps and misinterpretations that can arise in statistical analysis, a key component of Lean Six Sigma.
Debunking the ‘Average’ Myth
Pyzdek kicks off the discussion by tackling the concept of averages, revealing how they can often conceal as much as they reveal. The average salary in a company might mask wage disparities, as a few large incomes can drastically skew the average upward.
The Critical Role of Sampling Bias
He then explores the idea of sampling bias, a common statistical pitfall. The selection process can greatly influence the results of a study, as Pyzdek demonstrates with a simple coin flip experiment. Ensuring an unbiased sample is crucial to obtaining valid results in Lean Six Sigma.
Unveiling Simpson’s Paradox
An intriguing segment of the presentation revolves around Simpson’s Paradox. Pyzdek highlights how a trend seen in separate groups can reverse when the groups are combined, emphasizing the importance of considering all relevant variables when interpreting data.
The ‘Yippee’ Soda Saga: Unpacking Relative and Absolute Risk
One of the most compelling parts of Pyzdek’s talk involves a hypothetical soda named ‘Yippee.’ While drinking ‘Yippee’ doubles the relative risk of dying in a year, the absolute risk increase is fractional. The lesson? Always consider both relative and absolute risks to avoid misleading conclusions.
Piercing Through the Veil of Published Research
Lastly, Pyzdek brings the spotlight onto published research findings. He cautions that ‘peer-reviewed’ doesn’t always mean error-free, urging viewers to critically evaluate study methodologies and limitations before drawing conclusions.
Through this insightful presentation, Pyzdek arms us with the tools to navigate the fascinating yet complex world of statistics, a crucial aspect of Lean Six Sigma. His talk serves as a valuable reminder to challenge our understanding of numbers and to never take statistics at face value.
Leave a Reply