Your cart is currently empty!
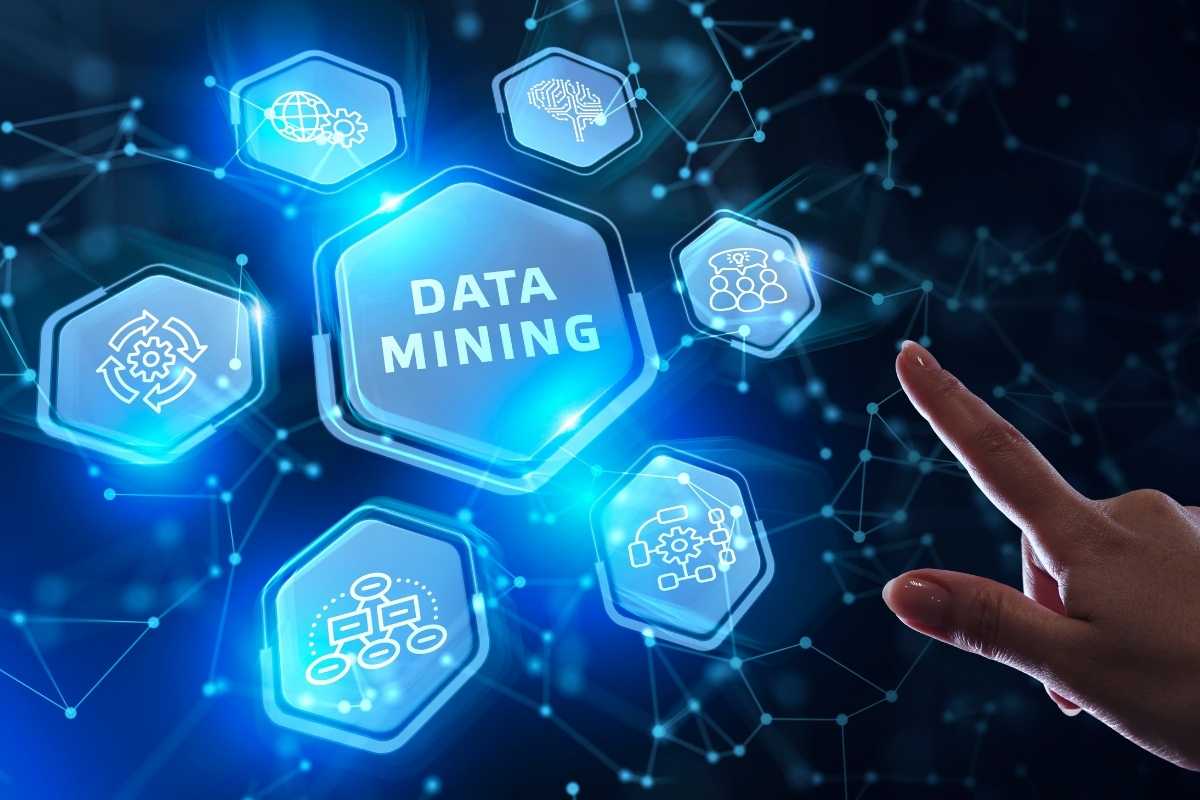
The Truth About DOE, Part 1
Design of experiments (DOE) and robust design of products are key parts of the Six Sigma process. To stay competitive, we need to offer great products to customers and we need to find and validate the optimum process settings to actually make the products. In short, we need a statistical model of our process.
However, as beneficial and productive as DOE is, the process of conducting a designed experiment has its drawbacks. The workplace, be it a factory, a retail establishment or an office, revolves around a routine. The routine is the “real work” that must be done to generate the revenues that keep the enterprise in existence.
Conversely, DOE is a disruption of the sacred routine. In an experiment, we intentionally change process factors all at once. The result is a statistical model that visualizes (repeat: visualizes!) the interactions, non-linearities and cause & effect relationships between what we can directly control (factors) and the results we want (responses). Often, the results are unpleasant and that’s why process settings weren’t changed in the first place. But lucky us, these unpleasantries help us understand our process nuances and create our statistical model.
The Six Sigma Paradox
Lean Six Sigma generates as much improvement by changing things as it does by reducing variability. It’s part of the Six Sigma paradox:
To reduce variability in products and processes, we must encourage variability and experimentation, at least on a temporary basis.
You mean we’ll intentionally make product that we can’t push out the door?! Yes, that’s right and it’s the only way to truly understand our process, reduce costs and maximize profits! The alternative is defeat by our competitors!
The Way Forward…Towards the Money
So what exactly should we do? Well, the first order of business is to NOT jump directly to a DOE. Instead, we must validate our measurement systems (outside the scope of this blog) and then do everything we can to increase the probability of DOE success. We need to get usable results the first time or cause future resistance to experimentation.
One thing we can do is squeeze useful insight from existing data. This “data mining” approach provides guidance to help us make better business decisions. However, it is likely that existing data were collected for a different purpose, so its typically used for guidance only. Fortunately, experience indicates existing data can help create a lean, well-considered DOE plan. For example, we can determine the range over which we may want to vary a process setting (pressure, pH, etc.) or we may find guidance on what factors we include in the experiment. Factor selection can be a daunting challenge and we need all the help we can get.
For clarity, in the absence of existing data, we’d typically collect and evaluate control chart data on experimental factors and responses before we begin the experiment. But that’s a subject outside the scope of this series of blogs.
The Data Mining Process & Useful Statistical Methods
Data mining is a process. Here is a data mining approach proven to work on many successful Lean Six Sigma projects
- Create a detailed inventory of data available throughout the organization. IT personnel may already have compiled this information. In many cases, a data logger is in place, but nobody was using the output.
- Determine the variables that apply to the process being improved and for which historical data exist.
- Create simple visualizations of the available data
- Discuss the visualizations in a collaborative environment
- Move on to more complex methods
- Continue collaborating
Meanwhile, here are a few statistical methods that are useful for data mining in the context of DOE prep. They have been proven to work well during many successful Lean Six Sigma projects.
- Involve operators and maintenance people (the data are in their heads!)
- I-MR control charts
- XBar-R control charts
- Fitted line plots
- Scatterplot matrices
- Predictive modeling (Neural Networks as a virtual DOE, CART® regression, etc.)
Control Charts for DOE Prep
The Pyzdek Institute has helped many clients troubleshoot designed experiments that went off the rails. We learned that a major cause of DOE disappointment was the incorrect assumption on the part of the DOE team that process factors and process responses were in statistical control prior to beginning the experiment. We therefore recommended experimenters precede designed experiments with a process review using control charts. Here is an example.
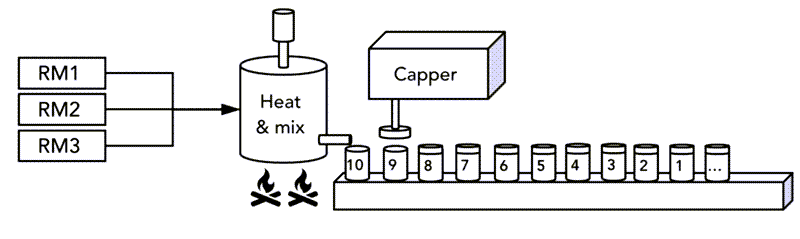
For this production line, we want to run a mixture + process DOE to determine the best ratio of Raw Material 1 (RM1), Raw Material 2 (RM2) and Raw Material 3 (RM3) and the optimum settings for temperature control and mixing speed. The goal is to optimize product characteristics that are critical to quality and customer satisfaction.
For this process, assume there is a previously-unknown data logger for the temperature controller recording a value at 9 am, 3 pm, 9pm and 3 am. We also have a supplier-provided control charts for the key properties of RM1 (a superb process improvement technique!). The use of control charts will get us through the first 4 steps of the data mining process and improve the probability of DOE success.
The Hunt for Special Cause Variation
To start, we create a control chart of recent data from the temperature data logger and review the charts in collaboration with others. This method alone provides excellent continuous improvement opportunity. In addition, team members should review recent supplier control charts. In both cases, special cause variation should be investigated and resolved before proceeding.
For this example, the team looked at data from January 1, 2022 to January 7, 2022 to simulate good SPC practices, i.e. establish useful limits and monitor data going forward.
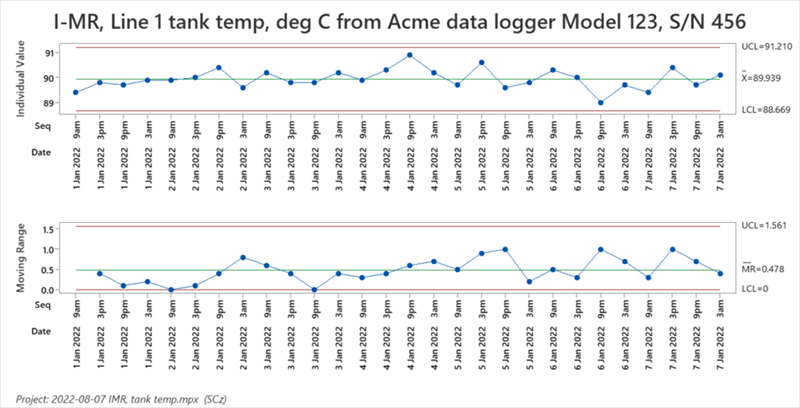
The control chart indicates no sign of special cause variation in the first 7 days worth of data. Keep with good practices, the data were reviewed every day for a few more weeks before running the experiment. IMPORTANT POINT: the presence of special cause variation in a process setting that is included in a DOE can seriously contaminate the predictive process model!
As a final note, there is no existing data for mixing speed, a DOE process factor. In this case, the DOE team should gather data in small, frequent samples and addresses any special cause variation they find BEFORE starting the experiment. For RM2 and RM3, the team should get the control chart data they need from their suppliers. WARNING: special causes in supplier processes can wreak havoc in a designed experiment.
Check out our SPC course to learn about sampling, constructing the right kind of control chart and the methods used to interpret them.
Next time, we’ll look at the use of Fitted line plots for DOE prep.
2 responses to “The Truth About DOE, Part 1”
-
Nice graphics, easy to read and easy to understand.
Very interestang subject. -
Thanks for stating an important fact, Tom. I’ve seen many examples of people jumping directly to a designed experiment without pausing to plan, collaborate, mine existing data and/or study process controls. In many cases, their statistical models were not useful to make important business decisions.
While I admire people’s enthusiasm for DOE, experimenters that don’t ease back and collect thoughts before running their plan can waste time, money and opportunity and set people against the idea of running experiments in the future.
Leave a Reply